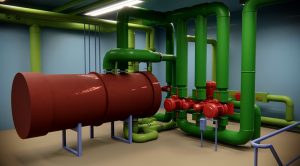
Digital Twins with Game Engines
A digital twin (Digital Twin) is a virtual model of a product, process, or service. It can be used to visualize the operation of the
A digital twin (Digital Twin) is a virtual model of a product, process, or service. It can be used to visualize the operation of the
As a software lab, FrostBit is not a traditional software development unit, although we have a web and mobile team in addition to the XR
Everyone who is familiar with software technology trends within past few years has surely met with the concepts of machine and deep learning as well